I Dataset Description
Here is a brief description of the datasets that are used in this book.
I.1 Alcohol disorders
In the book repo: alcohol-disorders.csv.
Share of males and females, suffering from alcohol use disorders (percentage of population). Alcohol dependence is defined by the International Classification of Diseases as the presence of three or more indicators of dependence for at least a month within the previous year. This is given as the age-standardized prevalence which assumes a constant age structure allowing for comparison by sex, country and through time.
IHME, Global Burden of Disease Study (2019) – processed by Our World
in Data.
Dowloaded from OWiD
Variables:
- Entity: country, only Argentina, Kenya, Taiwan, Ukraine and the U.S. are included.
- Code: 3-letter country code
- Year: 2015–2019 (only a subset of the original)
- disordersM: number of cases of alcohol use disorders per 100 people, in males, age-standardized
- disordersF: number of cases of alcohol use disorders per 100 people, in females, age-standardized,
- population: Population (historical estimates),
Example:
## # A tibble: 4 × 6
## country Code Year disordersM disordersF population
## <chr> <chr> <dbl> <dbl> <dbl> <dbl>
## 1 Taiwan TWN 2017 0.886 0.260 23665028
## 2 Kenya KEN 2017 0.742 0.658 48948140
## 3 Kenya KEN 2018 0.752 0.662 49953300
## 4 Taiwan TWN 2018 0.892 0.259 23726186
I.2 Babynames
R package babynames contains a dataset babynames. It includes ass baby names given in the U.S. between 1880-2017 at least 5 times each year for each sex. Data originates from U.S. Social Security Administration.
You can load it with library(babynames)
, that loads a single data
frame babynames
.
Example:
## # A tibble: 3 × 5
## year sex name n prop
## <dbl> <chr> <chr> <int> <dbl>
## 1 1991 M Detron 5 0.00000236
## 2 1973 M Klint 24 0.0000149
## 3 1957 M Delynn 10 0.00000457
Variables:
- year: 1880-2017
- name: the name
- sex: “F” or “M”
- n: how many babies got this name (withing year/sex)
- prop: proportion of babies who got this name in the given year (within year/sex).
I.3 Country-concept similarity
In the book repo: country-concept-similarity.csv.bz2. This dataset shows the similarity between country names and a set of different words, and it is calculated based on texts that were scraped from internet around 2015. The dataset looks like
## # A tibble: 2 × 12
## country terrorism nuclear trade battery regime volcano palm fir flood
## <chr> <dbl> <dbl> <dbl> <dbl> <dbl> <dbl> <dbl> <dbl> <dbl>
## 1 aruba 0.0891 -0.011 0.0504 -0.01 -0.0356 0.166 0.293 0.0965 0.0158
## 2 afghanistan 0.447 0.220 0.109 0.0578 0.180 0.129 0.116 0.129 0.159
## drought mountain
## <dbl> <dbl>
## 1 0.0581 0.107
## 2 0.160 0.161
One can see that “Afghanistan” and “terrorism” are much more similar (similarity 0.447) than e.g. “Afghanistan” and “trade” (similarity 0.109). We do not go into details here about how the similarity is measured, but broadly, it means how frequently are these words used in a similar context as the corresponding country names.
I.5 CS-GO
Dataset about CS-GO (video game) reviews: each line is a review. It is scraped from Steam website by mulhod, see the original repo at GitHub. The dataset is not really documented, but you can guess based on the column names.
Sample:
## # A tibble: 3 × 8
## rating nHelpful nFunny nScreenshots date hours nGames nReviews
## <chr> <dbl> <dbl> <dbl> <chr> <dbl> <dbl> <dbl>
## 1 Recommended 2 0 539 Oct 16, 2014, 3:30AM 903. 295 4
## 2 Recommended 2 0 54 Dec 28, 2013, 2:56AM 624. 51 6
## 3 Recommended 3 0 25 Aug 15, 2014, 5:00PM 615. 23 2
Variables:
- rating”: Recommended/Not recommended
- nHelpful”: number voted helpful
- nFunny”: number found funny
- nScreenshots”: number of screenshots
- date”: date posted
- hours”: total game hours by the reviewer
- nGames”: number of games
- nReviews”: number of reviews
TBD: anyone knows steam and can help here?
I.6 Diamonds
It is a built-in dataset in ggplot2 library, so it is already loaded when you load the library. It contains price, shape, color and other information for 53940 diamonds. A sample of it looks
## # A tibble: 5 × 10
## carat cut color clarity depth table price x y z
## <dbl> <ord> <ord> <ord> <dbl> <dbl> <int> <dbl> <dbl> <dbl>
## 1 0.31 Ideal H IF 62 56 772 4.36 4.39 2.71
## 2 2.01 Ideal H SI2 61.9 57 14167 8.09 8.06 5
## 3 0.52 Ideal E VVS2 61.8 56 2430 5.15 5.18 3.19
## 4 1.05 Ideal F SI1 61.3 54 5842 6.58 6.61 4.04
## 5 1 Very Good H SI2 63.4 61 3920 6.33 6.29 4
Variables:
carat: mass of diamonds in caracts (ct), 1 ct = 0.2g
cut: cut describes the shape of diamond. There are five different cuts: Ideal is the best and Fair is the worst in these data. Better cuts make diamonds that are more brilliant.
Note: cut is an ordered factor, see Section 18.2.3.
color: There are 7 color levels, J (no color) is the best and D the worst, any color hue is considered not desirable.
clarity: measures the defects in diamonds, IF (internally flawless) is the best, and I1 is the worst.
depth, table: measures of the diamond shape
price: in $
x, y, z: diamond size, mm
I.7 Fatalities
The U.S. Traffic fatalities by state in 1980’s. This is a subset of dataset Fatalities in the AER package. Example:
## # A tibble: 4 × 4
## year state fatal pop
## <dbl> <chr> <dbl> <dbl>
## 1 1984 OR 572 2675998.
## 2 1983 OR 550 2659999
## 3 1987 WA 780 4537997
## 4 1984 WA 746 4348992
Variables:
- year: 1982-1988
- state: only MN, OR, WA
- fatal: total number of traffic fatalities
- pop: population
I.8 Height-weight
In the book repo: height-weight.csv.
Synthetic dataset of five lines to demonstrate certain data properties. Here is the whole dataset, the meaning of columns is obvious:
## # A tibble: 5 × 4
## sex age height weight
## <chr> <dbl> <dbl> <dbl>
## 1 Female 16 173 58.5
## 2 Female 17 165 56.7
## 3 Male 17 170 61.2
## 4 Male 16 163 54.4
## 5 Male 18 170 63.5
I.9 Icecream
It is located in package Ecdat. It contains 30 four-weekly observations of ice cream consumption in 1950s in the U.S. Example:
## cons income price temp
## 18 0.443 78 0.277 72
## 13 0.329 86 0.272 32
## 21 0.319 85 0.292 44
## 9 0.269 76 0.265 32
Variables:
- cons: consumption of ice cream per head (in pints);
- income: average family income per week (in US Dollars);
- price: price of ice cream (per pint);
- temp: average temperature (in Fahrenheit);
I.10 Ice extent
TBD: an explanatory figure of area/extent
In the book repo: ice-extent.csv.bz2.
National Snow & Ice Data Center (NSIDC) data about sea ice extent and area. Downloaded from U Colorado
A sample of data:
## # A tibble: 5 × 7
## year month `data-type` region extent area time
## <dbl> <dbl> <chr> <chr> <dbl> <dbl> <dbl>
## 1 2014 11 Goddard N 10.1 8.75 2015.
## 2 2000 9 Goddard N 6.25 4.35 2001.
## 3 1991 1 Goddard S 5.34 3.52 1991.
## 4 1985 11 Goddard S 16.1 12.3 1986.
## 5 1986 7 Goddard S 15.3 12.1 1987.
I haven’t found description of the variables, but these are fairly
self-explanatory:
- year
- month: (1-12)
- data-type: looks like the name of the satellite or another info provider
- region: “N” for northern, “S” for southern hemisphere
- extent: sea ice extent, in M km2. Extent is the sea surface area where the ice concentration is at least 15%.
- area: sea ice surface area, M km2
- time: a continuous time variable, made of year and month \(\mathit{time} = \mathit{year} + \mathit{month}/12 - 1/24\). This describes roughly the middle of each month as measured in years.
I.11 Iris
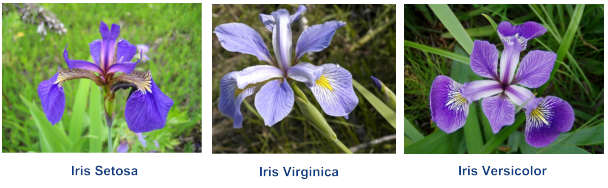
Iris flowers are beautiful. setosa, virginica and versicolor.
Iris dataset is collected by Ronald Fisher 1936. It contains sepal and petal measures of 150 iris flowers of species setosa, versicolor and virginica (50 of each). It is an R built-in dataset and does not even have to be loaded, you can just use variable iris.
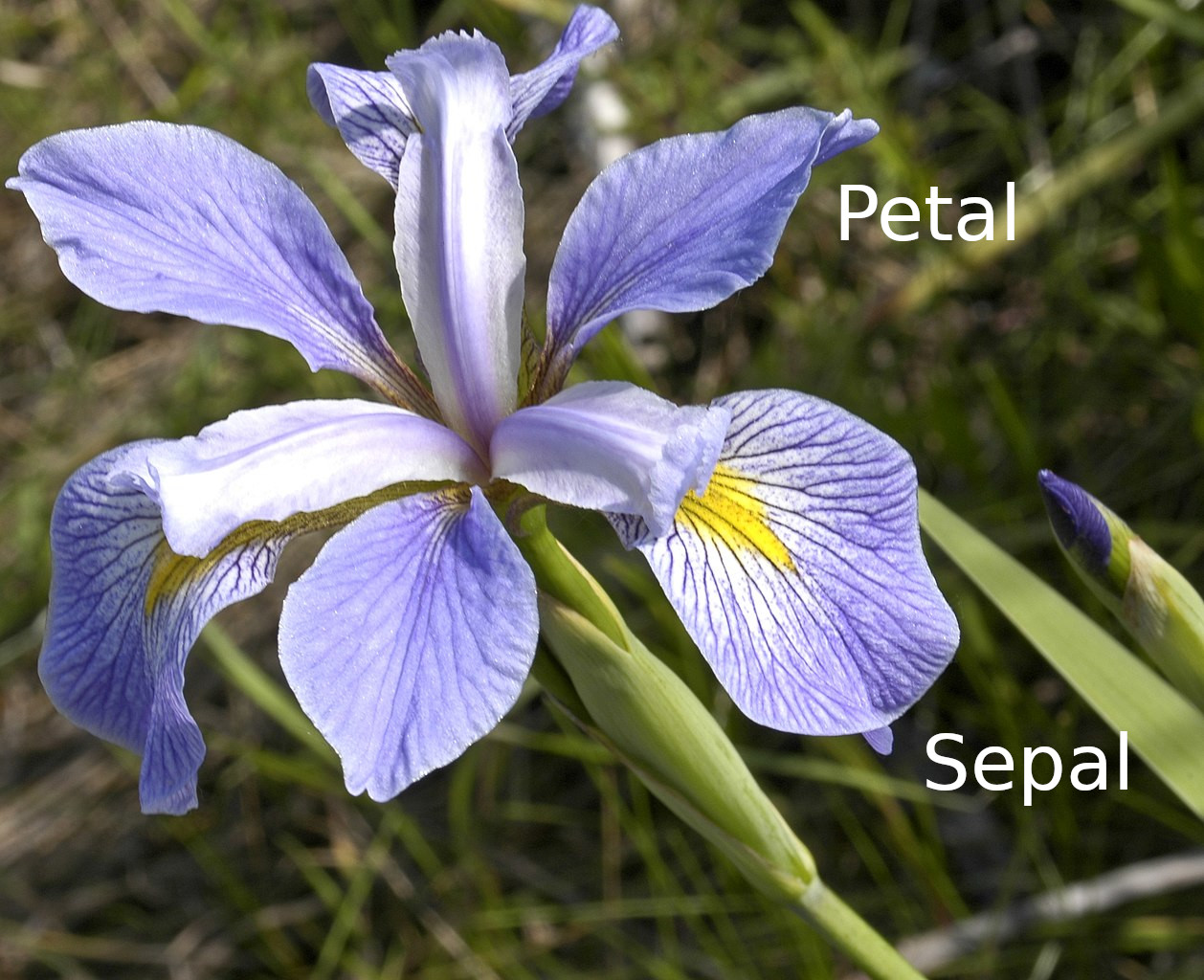
Petals and sepals are parts of the flower (virginica).
- Sepal.Length: sepal length, in cm
- Sepal.Width
- Petal.Length
- Petal.Width
- Species: setosa/versicolor/virginica
A small example of it:
## Sepal.Length Sepal.Width Petal.Length Petal.Width Species
## 1 6.4 2.8 5.6 2.1 virginica
## 2 7.7 3.8 6.7 2.2 virginica
## 3 4.7 3.2 1.3 0.2 setosa
## 4 5.0 3.4 1.5 0.2 setosa
I.12 Orange tree growth
It is an R built-in dataset, however, as that uses more complex data structures, a copy of it is in repo as a plain csv file: orange-trees.csv
Variables:- Tree: an ordered factor indicating the tree on which the measurement is made. The ordering is according to increasing maximum diameter.
- age: a numeric vector giving the age of the tree (days since 1968/12/31)
- circumference: a numeric vector of trunk circumferences (mm). This is probably “circumference at breast height”, a standard measurement in forestry.
I.13 US States
R has multiple small datasets about the US states. They are built-in variables, so you do not need to do anything special to load these. Examples:
## [1] "Alabama" "Alaska" "Arizona" "Arkansas" "California"
## [1] "AL" "AK" "AZ" "AR" "CA"
Importantly, all these vectors contain data in the same order, so you can use names to find the value for the corresponding state.
I.14 Titanic
In repo as titanic.csv.bz2.
List of RMS Titanic passengers, their name, age and some more data, and whether they survived the shipwreck. It was collected by the investigation committee, and contains most of the passengers on the boat. The dataset is available in various sources, e.g. at kaggle. The variables are
- pclass: Passenger Class (1 = 1st; 2 = 2nd; 3 = 3rd)
- survived: Survival (0 = No; 1 = Yes)
- name: Name
- sex: Sex
- age: Age
- sibsp: Number of Siblings/Spouses Aboard
- parch: Number of Parents/Children Aboard
- ticket: Ticket Number
- fare: Passenger Fare
- cabin: Cabin
- embarked: Port of Embarkation (C = Cherbourg; Q = Queenstown; S = Southampton)
- boat: Lifeboat code (if survived)
- body: Body number (if did not survive and body was recovered)
- home.dest: The home/final destination of passenger
A small example of it:
pclass | survived | name | sex | age | sibsp | parch | ticket | fare | cabin | embarked | boat | body | home.dest |
---|---|---|---|---|---|---|---|---|---|---|---|---|---|
2 | 1 | Bryhl, Miss. Dagmar Jenny Ingeborg | female | 20 | 1 | 0 | 236853 | 26.0000 | NA | S | 12 | NA | Skara, Sweden / Rockford, IL |
3 | 0 | Vande Velde, Mr. Johannes Joseph | male | 33 | 0 | 0 | 345780 | 9.5000 | NA | S | NA | NA | NA |
3 | 0 | Peduzzi, Mr. Joseph | male | NA | 0 | 0 | A/5 2817 | 8.0500 | NA | S | NA | NA | NA |
3 | 1 | Andersen-Jensen, Miss. Carla Christine Nielsine | female | 19 | 1 | 0 | 350046 | 7.8542 | NA | S | 16 | NA | NA |
I.15 Ukraine’s regional population
In repo as ukraine-oblasts-population.csv. Copied from the Wikipedia table 2024-03-03. Population as of 2015.
Example:
## # A tibble: 3 × 4
## Prefecture Population `Urban population` `Rural population`
## <chr> <dbl> <dbl> <dbl>
## 1 Donetsk Oblast 4387702 3973317 414385
## 2 Dnipropetrovsk Oblast 3258705 2724872 533833
## 3 Kyiv 2900920 2900920 NA
The variables are self-explanatory.
I.16 Ukraine with regions
In repo as ukraine-with-regions_1530.geojson. The national borders and regional (oblast) borders of Ukraine in geojson format. Provided by Cartography Vectors.
The map:library(sf)
library(ggplot2)
map <- read_sf("data/ukraine-with-regions_1530.geojson")
ggplot(map) +
geom_sf()
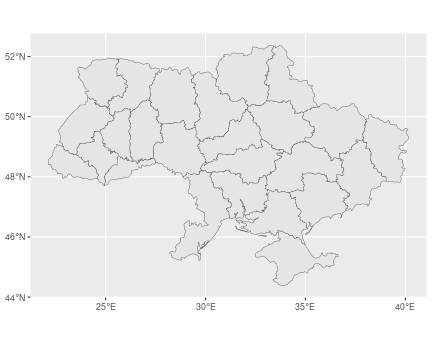
National and regional (oblast) borders of Ukraine. Provided by Cartography Vectors.