Ion Mobility-Mass Spectrometry (IM-MS)
Ions formed from different classes of biomolecules adopt different conformations in the gas phase, with some being more densely packed while others being more loosely packed depending on their intramolecular physical property. It was previously demonstrated that the packing efficiency of biomolecular ions decreases in the order of lipids < peptides < carbohydrates < oligonucleotides, which suggest that for the same m/z value, lipids display the largest collision cross section (CCS) [1]. This IM characteristics of lipids make these ions stand out of the complex molecular matrix that can be formed from a biological system. The Figure below illustrates such separation of biomolecule classes.
Even within the lipids, some sub-classes can be separated [2, 3]. For example, sphingomyelins (SMs) display larger CCSs than phosphatidylcholines (PCs) even though both of them have the same headgroup.
Calibration of CCS in Traveling Wave Ion Mobility-Mass Spectrometry (TWIM-MS)
Unlike drift tube ion mobility (DTIM) analysis, a precise relationship between CCS and the drift time in TWIM has not been established. Thus, in order to determine CCS values using TWIM, calibration of the CCS vs. drift time relationship using analytes with known CCS values is necessary. Previous reports and our work suggested that calibration using ions with similar physical properties is desirable in order to achieve the highest CCS accuracy. For lipidomics studies, we have established a series of phospholipid calibrants, including phosphatidylethanolamine (PEs) and phosphatidylcholines (PCs), for the measurement of lipid CCS values using TWIM. These phopholipid calibrants perform the best in comparison with other types of calibrants that occupy distinctly different conformational space in the drift time-m/z plot, such as poly-alanines (PolyAla), tetraalkylammonium salts (TAA), and hexakis(fluoroalkoxy)phosphazines (HFAP) [4, Hines et al. Anal. Chem., 2016].
With this calibration strategy, we have measured over 400 lipid CCS values so far (see Hines et al. J. Lipid Res., 2017 and Hines et al. mSphere, 2017). As seen below, different lipid classes occupy distinct conformational space in the CCS-m/z 2D plot.
Metabolomics and Lipidomics by Liquid Chromatography-Ion Mobility-Mass Spectrometry (LC-IM-MS)
We aim to develop both targeted analysis of small molecules using tandom mass spectrometry (MS/MS) and untargeted analysis of the metabolome using LC-IM-MS. The unique advantages of LC-IM-MS are: a) three-dimensional data allows more definite identification of an ion with retention time, CCS, and m/z; b) remove isobaric ions from the ions of interest by IM separation, and c) post-mobility fragmentation provides additional structural information of ions. The Figure below illustrates the typical workflow. Based on this strategy, we have established a comprehensive lipidomics method by coupling hydrophilic interaction liquid chromatography (HILIC) with IM-MS (Hines et al. J. Lipid Res., 2017).
Structural Characterization of Drugs and Drug Metabolites
Drugs are known for their chemical diversity as they bind to various enzyme targets and receptors. Taking advantages of the rapid nature of IM separation and the CCS values reflecting gas-phase structure, we are developing high-throughput and high-confidence identification of drug molecules from biological matrixes, pharmaceutical formulations, and contaminants using IM-MS. Recently, we measured CCS values of 1425 drug or drug-like molecules using a high-throughput flow injection-IM-MS method (Hines et al. Anal. Chem., 2017). Overall drug distribution in the CCS-Mass plot suggests large structural diversity of these molecules in the gas phase (Figure 1 – gray background), but a tight structure−function relationship was observed for each class of drugs with a specific target (Figure 1 – colored). Some drugs display multiple distinct conformations in the gas phase driven by protonation sites (protomers) (Figure 2). We aim to adapt such strategy for the studies of drug metabolites. Check out our related publications below by Hines et al. and Ross et al.
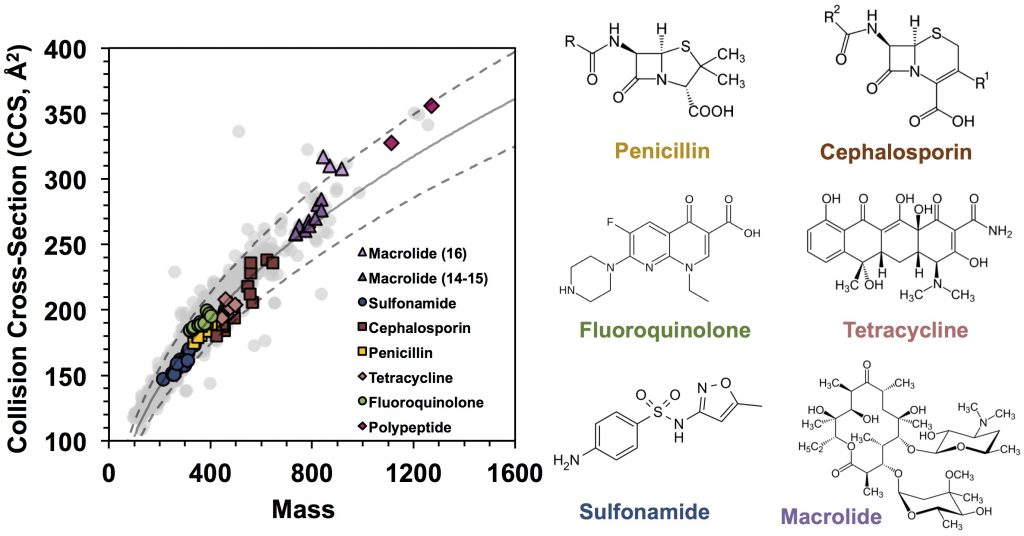
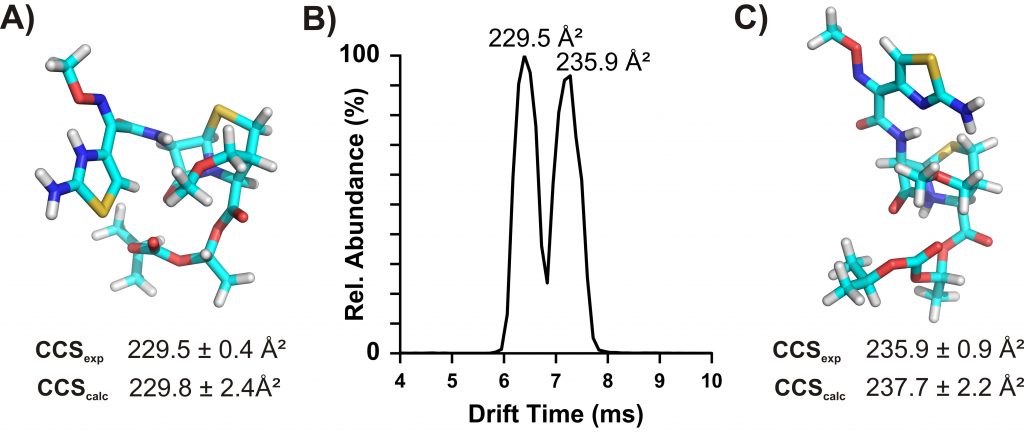
Check out our latest publications on lipidomics, characterization of drugs an drug metabolites, and the development of machine learning-based models for the prediction of CCS values.
References
1. Fenn, L. S.; Kliman, M.; Mahsut, A.; Zhao, S. R.; McLean, J. A. Anal. Bioanal. Chem. 2009, 394, 235.
2. Kliman, M.; May, J. C.; McLean, J. A. Biochim. Biophys. Acta, Mol. Cell Biol. Lipids 2011, 1811, 935.
3. May, J. C.; Goodwin, C. R.; Lareau, N. M.; Leaptrot, K. L.; Morris, C. B.; Kurulugama, R. T.; Mordehai, A.; Klein, C.; Barry, W.; Darland, E.; Overney, G.; Imatani, K.; Stafford, G. C.; Fjeldsted, J. C.; McLean, J. A. Anal. Chem. 2014, 86, 2107.
4. Hines, K. M., May, J. C., McLean, J. A., Xu, L.* (2016) Evaluation of Collision Cross Section Calibrants for Structural Analysis of Lipids by Traveling Wave Ion Mobility-Mass Spectrometry, Chem. 88, 7329–7336.
5. Hines, K. M., Herron, J., Xu, L.* (2017) Assessment of Altered Lipid Homeostasis by HILIC-Ion Mobility-Mass Spectrometry-Based Lipidomics. J. Lipid Res. 58, 809-819.
6. Hines, K. M.; Waalkes, A.; Penewit, K.; Holmes, E. A.; Salipante, S. J.; Werth, B. J.; Xu, L.* (2017) Characterization of the Mechanisms of Daptomycin Resistance Among Gram-Positive Bacterial Pathogens by Multi-Dimensional Lipidomics. mSphere, 2, e00492-17.
7. Hines, K. M.; Ross, D. H.; Davidson, K. L.; Bush, M. F.; Xu, L.* (2017) Large-Scale Structural Characterization of Drug and Drug-Like Compounds by High-Throughput Ion Mobility-Mass Spectrometry. Anal. Chem. 89, 9023-9030.
8. Hines, K. M., and Xu, L.* (2019) Lipidomic consequences of phospholipid synthesis defects in Escherichia coli revealed by HILIC-ion mobility-mass spectrometry, Chem. Phys. Lipids, 219, 15-22.
9. Ross, D. H.; Seguin, R. P.; Xu, L.* (2019) Characterization of the Impact of Drug Metabolism on the Gas-Phase Structures of Drugs Using Ion Mobility-Mass Spectrometry. Analytical Chemistry, 91, 14498-14507.
10. Ross, D. H.; Cho, J. H.; Xu, L.* (2020) Breaking Down Structural Diversity for Comprehensive Prediction of Ion-Neutral Collision Cross Sections. Anal. Chem., 92, 4548-4557.
11. Ross, D. H.; Cho, J. H.; Zhang, R.; Hines, K. M.; Xu, L.* (2020) LiPydomics: A Python Package for Comprehensive Prediction of Lipid Collision Cross Sections and Retention Times and Analysis of Ion Mobility-Mass Spectrometry-Based Lipidomics Data, Anal. Chem., 92, 14967–14975.
12. Ross, D. H.; Xu, L.* (2021) Determination of drugs and drug metabolites by ion mobility-mass spectrometry: a review, Anal. Chim. Acta, 1154, 338270. Doi: 10.1016/j.aca.2021.338270.