About Me
I am an Associate Professor in the Information School at the University of Washington, an affiliate faculty at the Paul G. Allen School of Computer Science & Engineering and the Founding Co-Director of RAISE, a Center for Responsibility in AI Systems and Experiences.
My research interests are in Social Computing and Responsible AI and my work sits at the intersection of Computer Science and Social Science. As an interdisciplinary scholar, I draw from a range of methods from the fields of human computer interaction, large-scale data analytics, machine learning, and AI to understand human behavior online and better support human-human and human-machine communication.
From August 2017 to 2020, I was an assistant professor in the Department of Computer Science at Virginia Tech. Before that I got my PhD in Computer Science from Georgia Tech.
Current Research Topics
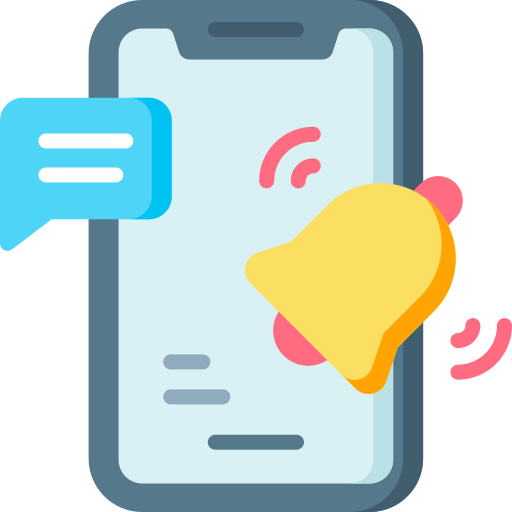
Generative and Responsible AI
Generative AI is rapidly transforming our information space. It has allowed the capability to mass produce biased and problematic information at unprecedented scale and speed, thus raising new trust and safety issues and posing new challenges for our online information space. How do we ensure that genAI tools produce socio-demographically diverse, value-aligned, and responsible data? How has AI changed what kind of misinformation or disinformation is spread online? What are the new opportunities and epistemic challenges generative AI technology is posing in the domain of health, journalism, fact-checking and others? We are currently exploring answers to these questions, some in collaboration with external stakeholders.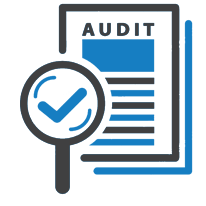
Auditing and Contesting Algorithmic Decisions
There is a growing concern that problematic content online are often amplified by the very algorithms driving the online platforms. Yet, their opacity makes it impossible to determine how and when algorithms are amplifying such content. How do we advance responsibility in algorithm design? How do we advance responsibility in algorithm design? My lab is conducting computational audits to determine the adverse effects of algorithms. We have conducted the first systematic misinformation audit on YouTube to empirically establish the “misinformation filter bubble effect”, followed by auditing an e-commerce platform to reveal how algorithms amplify vaccine misinformation. We are also interested in questions around contestability in large scale online systems.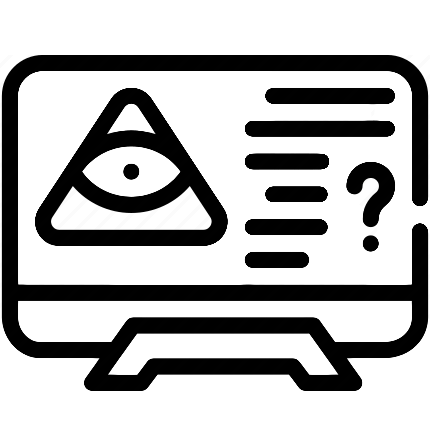
Understanding Problematic Phenomena
Today, online social systems have become integral to our daily lives. Yet, these systems are now rife with problematic content, whether they be harmful misinformation, damaging conspiracy theories, or violent extremist propaganda. Left unchecked, these problems can negatively impact our democracy and society at large. My lab has been investigating what makes people join online conspiratorial communities, how dramatic events effect conspiratorial discussions, what are the narrative motifs of these discussions. We have also looked at online extremism and have answered questions ranging from how hate groups frame their hateful agenda to what roles they play.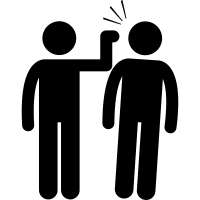
Designing to Defend Against Problematic Information
Our lab is also interested in designing systems to counter problematic information. Checkout our work on OtherTube and NudgeCred. NudgeCred is a socio-technical intervention powered by the idea of nudges, choice preserving architectures that steer people in a particular direction, while also allowing them to go their own way. We have just started delving into questions around designing trustworthy journalism. How can news organizations effectively demonstrate to the public the key aspects of good journalism, the primary features that make a story trustworthy, and the core aspects that govern the production and reporting of a news story?Recent Publications (all)
-
Online Myths on Opioid Use Disorder: A Comparison of Reddit and Large Language Model
S. Mittal, H. Jung, M. ElSherief, T. Mitra, M. De Choudhury | ICWSM 2025 | paper | doi
-
Online Myths on Opioid Use Disorder: A Comparison of Reddit and Large Language Model
H. Jung, P. Juneja, T. Mitra | ICWSM 2025 | paper | doi
-
Social Dynamics and Mobilization Potential of Online Election Narratives
K. Engel, T. Mitra, E. Spiro | ICWSM 2025 | paper | doi
-
They are uncultured: Unveiling Covert Harms and Social Threats in LLM Generated Conversations
P. Dammu, H. Jung, A. Singh, M. Choudhury, T. Mitra | EMNLP 2024 | paper | doi
-
ValueScope: Unveiling Implicit Norms and Values via Return Potential Model of Social Interactions
C. Park, S. Li, T. Mitra, H. Jung, S. Volkova, D. Jurgens, Y. Tsvetkov | EMNLP 2024 | paper | doi