Browse this page if you are interested in hearing about my ongoing and past research projects.
RHESSys-WMFire allows us to explore interactions among climate, vegetation, fuels, and wildfire
RHESSys-WMFire is a coupled model of spatially distributed ecohydrology, wildfire spread, and fire effects. It is the result of a long-standing collaboration I have had with the Tague lab at UC Santa Barbara. This model system tracks the water and nutrients that move through the watershed, from the precipitation that lands on vegetation and soils, then is transpired through the plants, evaporated through surfaces, or soaks into the ground and eventually ends up in the stream. Plants intercept sunlight, photosynthesize, and grow, depending in part on water availability. Dead plant material falls to the ground and provides fuel for potential wildfire. This fuel is lost either through decomposition or combustion. This model system is unique because we can explore not only how climate affects wildfire within one season (e.g., a hotter and drier summer is likely to have more wildfire), but also how it affects vegetation growth and therefore the production of fuels. Wildfire spread depends on the fuel loading and how dry the fuels are. Fire effects also depend on fuel loading, dryness, and the vertical continuity of the fuels. In this sense the model is fully coupled–climate affects vegetation growth, vegetation makes fuels for wildfire, climate affects fire weather, wildfire affects vegetation.
Our ability to capture these feedbacks has resulted in interesting insights about climate-wildfire interactions. See Hanan et al. (2021) and Kennedy et al. (2021) for recent results. We are continuing to refine and improve the model as it is being applied to watersheds throughout the western US.
Spatially explicit analysis of fuels management improves our understanding of how we can restore fire-resilient forests
Time and again, when comparing a stand with a fuel reduction treatment to a nearby stand without, that the fuel treatment experienced less severe fire effects. This binary comparison has been helpful to demonstrate the effectiveness of fuel treatments (usually a mechanical thin combined with prescribed fire works best) to modify fire behavior and restore resilient forest structures. Such an analysis is limited, however, because we are also concerned with patterns across entire landscapes. Wildfire is an inherently contagious spatial process–what happens in a treated stand will depend a lot on what the fire is doing outside of that stand. By explicitly including these spatial relationships in our empirical analysis of wildfire burn severity, we can expand our understanding of how fuel treatments interact with wildfires.
We have looked at stand-level spatial patterns of burn severity as the wildfire moved from untreated to treated areas, and found that the distance into the treated area that high severity fire effects were experienced depended both on the post-treatment stand structure and on the intensity of the fire in the untreated area as it burned into the treated area. This analysis can inform how large a fuel treatment should be to achieve desired reductions in fire severity.
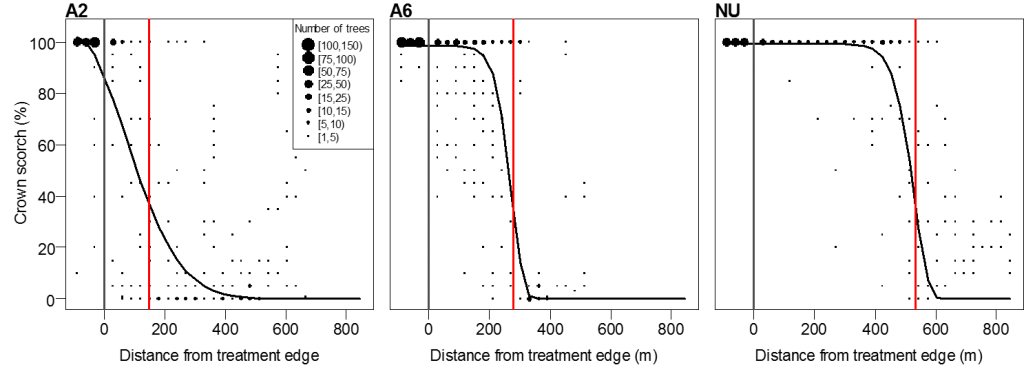
We are also concerned with landscape-scale fuel treatment effects. Satellites data can be used to map wildfire severity across an entire burned area, enabling detailed analysis of how landscape features and fuel treatments can predict fire severity. These data exhibit strong spatial autocorrelation and require spatially explicit analysis to take full advantage of the information contained therein. We use these techniques to ensure that we achieve credible statistical inference, but they also revealed that the spatial autocorrelation explains almost all of the variability in burn severity. Even after accounting for significant variables like topography, weather, and fuels, the easiest way to predict the severity of one pixel is by the severity of its neighbors.
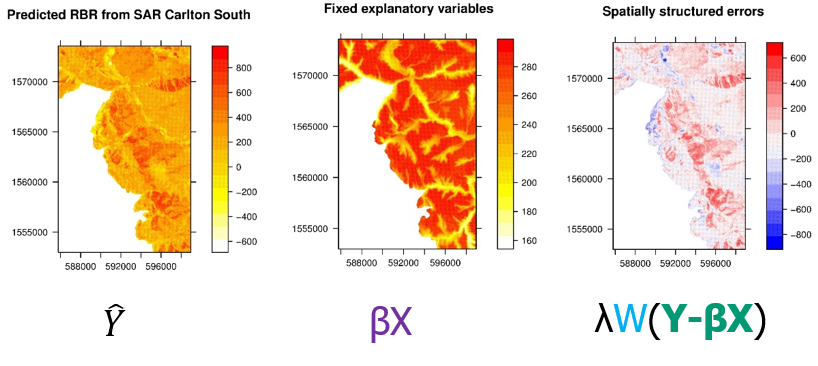
Wildfire is a strongly spatially contagious process and the spatial pattern is not explained by spatially structured predictors. We’re beginning to design empirical and simulation studies that explore how the structure of the spatial contagion itself may be explained by landscape-scale patterns. For example, does the strength of autocorrelation in burn severity change with different topographic complexities or patterns in fuel structures or continuity?
Resilient forests may require postfire fuels management
A consequence of the unusually high continuity of fuels in dry forests is the resulting postwildfire landscape of unusually large, contiguous patches of high severity wildfire. These landscapes have implications for what occurs when the area burns again (a reburn). Subsequent fire spread and fire effects tend to be limited for around 10 years or so after the initial wildfire–in this sense fire can be self-limiting. After that time of fire-related fuel limitation, reburn severity tends to follow the severity of the original burn. If an area burned initially at low severity, it is more likely to reburn at low severity. If it burned initially at high severity, it is more likely to reburn at high severity.
What to do with large high severity patches is a pressing and controversial question in fire management. There are costs and benefits of fuel treatments following large high severity fires. Whether, when, and where to use fuel treatments is unclear, as are the effective prescriptions that balance multiple management objectives (e.g., fuels management, wildlife habitat, water quality and yield, erosion control, etc.). What is clear is that we require credible studies to evaluate the consequences of postwildfire fuels management and to give credible data to guide management decisions.
A colleague with the US Forest Service works closely with managers in national forests across the western US who are grappling with theses questions. Together we are designing empirical field experiments and simulation experiments to evaluate alternative postfire treatment prescriptions relative to untreated control. For example, we can compare potential future fuel loadings between stands that have been treated and those that have not. We are also evaluating models that are commonly used for these decisions and their limitations. We have found that predictions of future fuel loads are particularly sensitive to decomposition rate, which could be impacted by climate change.
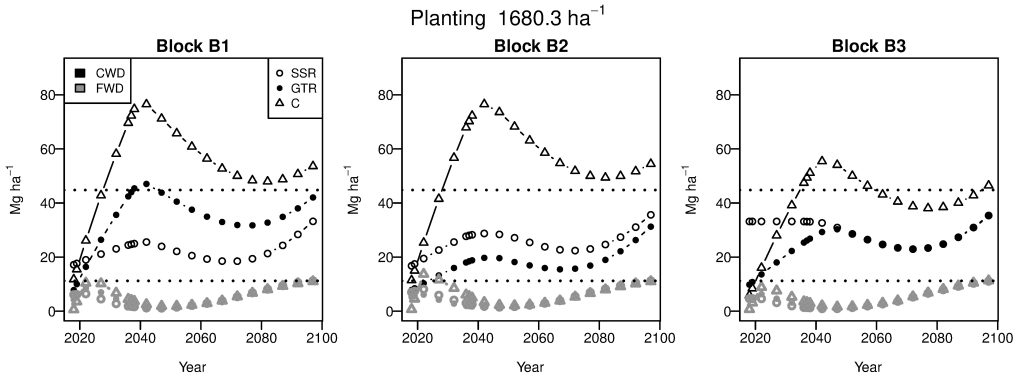