Data-driven inference of network connectivity for modeling the dynamics of neural codes
Front. Comp. Neuroscience 2014
Eli Shlizerman, Jeffrey A. Riffell and J. Nathan Kutz
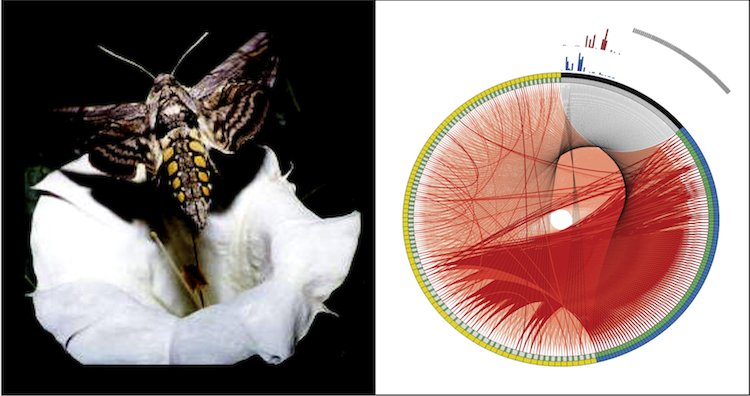
Summary
The olfactory system in insects is capable to encode scents into robust patterns of neural activity. This behavior is remarkably successful even in a noisy and dynamic environment. What is the connectivity within the olfactory neural network that produces such robust codes? To answer this question, we developed a method that is capable to recover the potential wiring of the antennal lobe, primary olfactory processing center in insects, from electrophysiological multi-neuron recordings. Our results show that inhibitory connections within the antennal lobe have a significant impact on the ability of the network to produce neural codes. Furthermore, we reveal wiring configurations that are capable to filter noise and enhance the contrast of the neural codes.Project Material
Paper, YouTube videosResults
Olfactory Processing
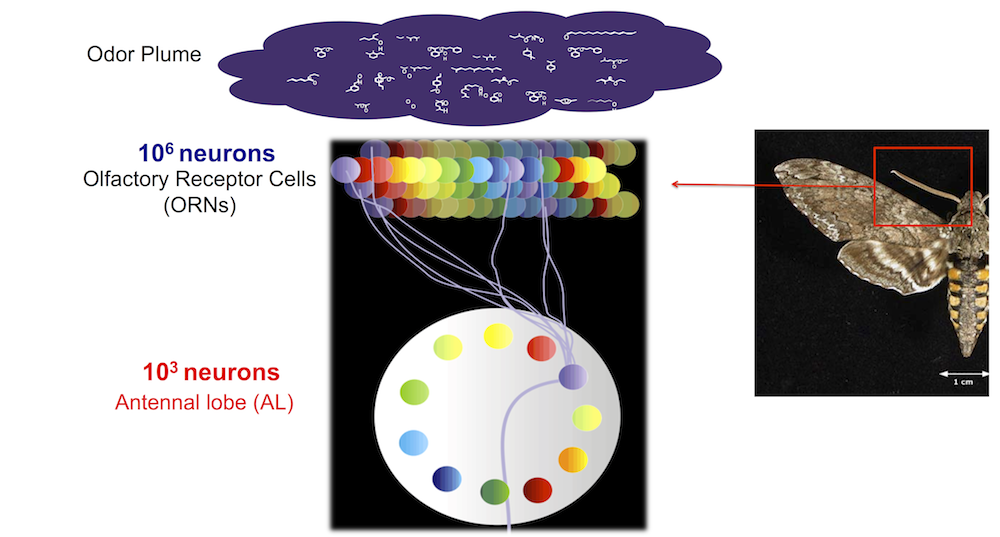
Moths sense chemicals with their antennas. On the antennas there are about a million of receptor neurons (ORNs) tuned to sense different chemicals (marked by different colors on the left). The receptors connect to the Antennal Lobe (AL) where there are much less neurons, about a thousand, and they are ordered in neuropils (Glomeruli). The Antennal Lobe codes stimuli into dynamical neural codes.
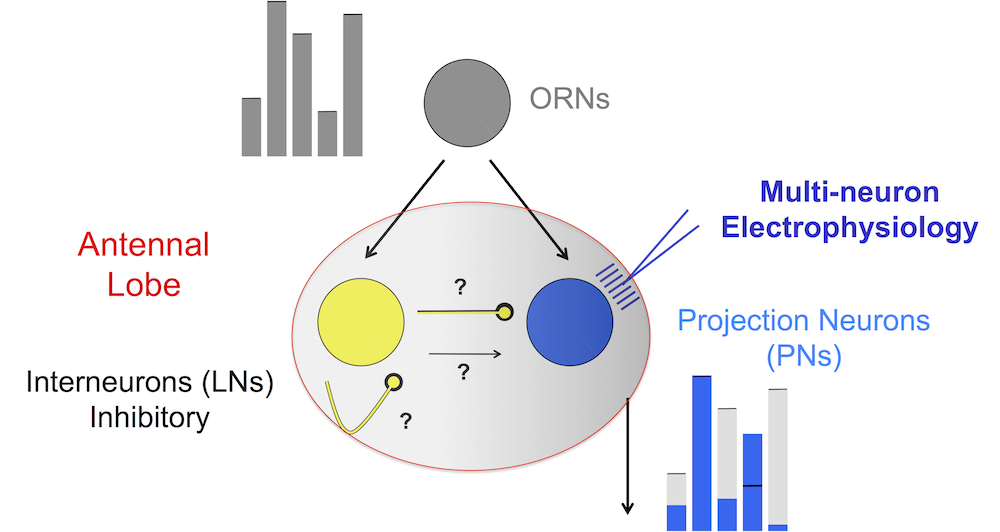
Both projection neurons (PNs) and interneurons (LNs) receive input from receptors. The LNs are mainly inhibitory. Via lateral inhibition LNs shape the response of PNs into neural codes, i.e., enhance the contrast of firing-rate patterns associated with the stimulus. The main challenge for modeling is that the lateral connections between LNs and PNs are unknown.
Neural Codes Library
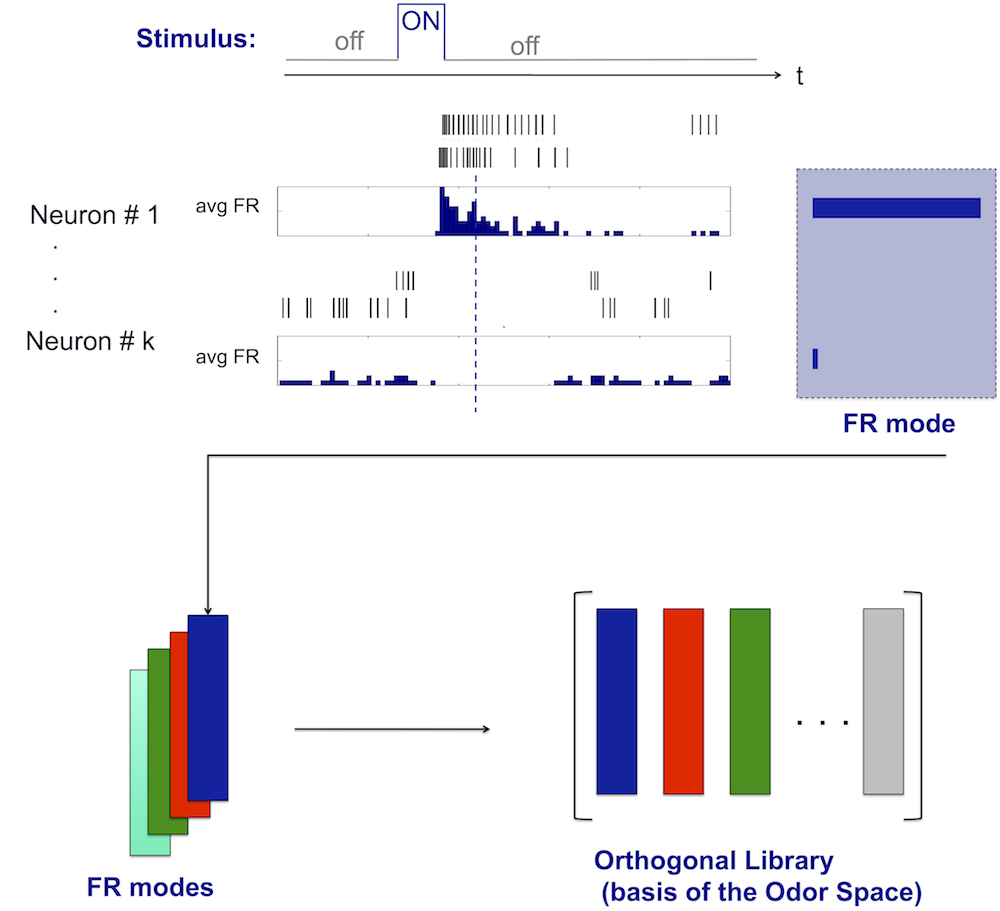
Projection of recorded data onto the library can be used as a recognition tool for the applied stimulus:
Data-driven Modeling
We use the library to model the wiring of inhibitory (LN) neurons that participate in the processing of tested stimuli.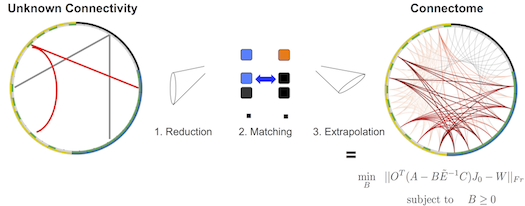
The wiring is determined by an automatic optimization routine that we have developed.
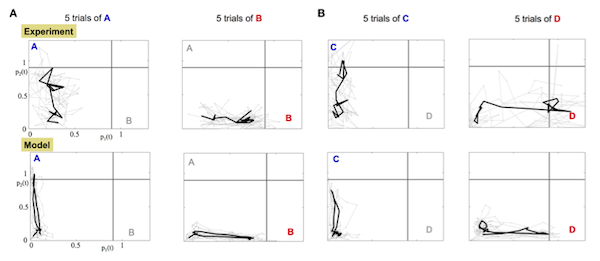
Acknowledgement
We thank the National Science Foundation Grant No. NSF/NIGMS DMS-1361145 (Eli Shlizerman and Jeffrey A. Riffell) and the University of Washington Royalty Research Fund (Jeffrey A. Riffell) for supporting the work. The authors would also like to acknowledge the partial support by the Department of Applied Mathematics and the Department of Biology at the University of Washington.Contact
For more information please contact:Eli Shlizerman (Data-Driven Dyn. Sys. Group, PI) and Jeff Riffell (Riffell Lab, PI)